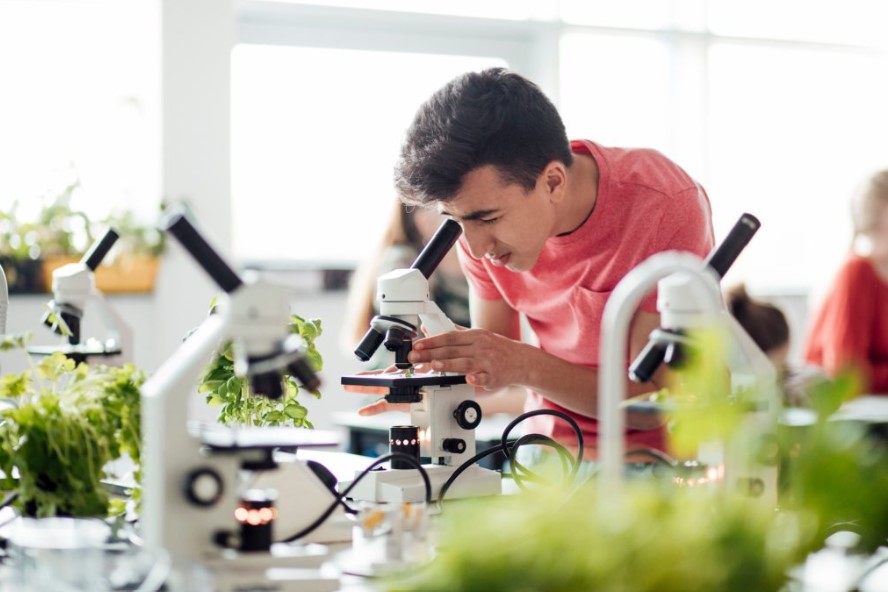
In the healthcare context, the amount of patient data being generated continues to balloon with every passing year. Such huge swaths of data, unimaginable a few decades ago, have the power to transform the future of the life sciences industry and improve patient outcomes.
Defining Real World Data (RWD)
According to the FDA, real world data are the data relating to patient health status and/or the delivery of health care routinely collected from a variety of sources.
Real world data can be gleaned from a handful of different sources. The FDA notes that these may include:
- electronic health records (EHRs)
- claims and billing activities
- product and disease registries
- patient-generated data
- data gathered from other sources that can inform on health status, such as mobile devices
Real world data is used to generate what’s known as real world evidence (RWE). These terms are closely related, but not interchangeable. According again to the FDA, real world evidence is “the clinical evidence regarding the usage and potential benefits or risks of a medical product derived from analysis of RWD”.
Real world data gives us real world evidence, which can be used, for example, to understand the effects of specific treatments on populations that are not represented in typical clinical trials, or to analyze long-term effects of drugs that are only studied for short periods during the trial phase
How real world data is changing the life sciences industry
Real world data, which in turn can be used to generate real world evidence, is set to have a disruptive impact on the life sciences industry.
A few applications of real world evidence in the life sciences field include:
Post-market observational studies
Though disease therapies that reach the market go through rigorous clinical trials before achieving approval, there is still more to be learned once the drug comes to market. RWE allows for the long-term study of a therapy and its effects on real patient populations many years after coming onto the market.
Precision medicine
Precision medicine is a topic that’s garnered a lot of interest over the past few years as more advanced data collection and analysis methodologies have made it increasingly viable. Precision medicine involves development of medical treatments that are tailored to specific populations of patients based on factors like genetics, lifestyle, and environment. RWD allows drug makers to analyze the effects of their therapies on these specific subgroups of the population who are not necessarily available to be studied in the trial phase.
RWD is essential to what’s known as comparative effectiveness — basically how effective is a drug in one population subgroup versus another? This information helps physicians make better decisions in treating their patients. If the physician knows that Drug A is shown by RWE to be more effective than Drug B for the patient’s specific subgroup, he or she can make a better choice in prescribing for that patient.
Contributing to clinical trials
The cost of bringing a new drug to market is enormous — an estimated $2 billion. Research can take a decade or more, and the majority of trials end in failure. RWD can be used to make more informed decisions about trial design. For example, in the case of designing drugs to treat rare diseases where trial candidates may be difficult to locate. RWD can help identify and recruit patients, indicating at an early stage whether the trial is feasible or not. Better trial design — and faster identification of unsuccessful trials — means effective therapies may be able to reach the market faster.
Current challenges surrounding RWD
Though RWD holds great promise for the future of the life sciences industry, it is not without challenges and limitations.
The biggest challenge surrounds the data itself. When data is pulled from a variety of sources there are often problems collating it within a unified, workable format. What’s more, data derived from clinical trials is standardized and reliable, whereas there may be accuracy issues with data derived from real world sources. Ensuring data validity is key to making the use of RWD safe and effective in its applications.
Inevitably, there is also structural resistance to change when it comes to RWD and RWE. Internal knowledge and understanding of when, how, and where RWD can benefit the organization is still a problem in many cases.
Conclusion
We’re living in an increasingly data-driven world, and in few places does data have more power to transform operations than in the life sciences industry. In the future, real world data will contribute to the development of more effective treatments while also reducing the costs of this development.